BrainChip is the worldwide leader in on-chip edge AI processing and learning technology, that enables faster, efficient, secure, and customizable intelligent devices untethered from the cloud. The company’s first-to-market neuromorphic processor, AkidaTM, mimics the human brain, the most efficient inference and learning engine known, to analyze only essential sensor inputs at the point of acquisition, executing only necessary operations and therefore, processing data with unparalleled efficiency and precision. This supports a distributed intelligence approach keeping machine learning local to the chip, independent of the cloud, dramatically reducing latency, while simultaneously improving privacy and data security.
The Akida neural processor is designed to provide a complete ultra-low power Edge AI network processor for vision, audio, smart transducers, vital signs and, broadly, any sensor application.
BrainChip’s scalable solutions, that can be used standalone or integrated into systems on chip to execute today’s models and future networks directly in hardware, empowering the market to create much more intelligent, cost-effective devices and services universally deployable across real-world applications in connected cars, healthcare, consumer electronics, industrial IoT, smart-agriculture and more, including use in a space mission and the most stringent conditions.
BrainChip is the foundation for cost-effective, fan-less, portable, real-time Edge AI systems that can offload the cloud, reducing the rapid growth in carbon footprint of datacenters. In addition, Akida’s unique capability to learn locally on device also reduces retraining of models in the cloud whose skyrocketing cost is a barrier to the growth of AIoT.
Interview with Nandan Nayampally, CMO at BrainChip.
Easy Engineering: What are the main areas of activity of the company?
Nandan Nayampally: BrainChip is focused on AI at the Edge. The vision of the company is to make AI ubiquitous. Therefore, the mission for the company is to enable every device to have on-board AI acceleration, the key to which is extremely energy-efficient, and yet performant neural network processing. The company has been inspired by the human brain – the most efficient inference and learning engine to build neuromorphic AI acceleration solutions. The company delivers this as IP which can be integrated into customers’ System on Chip (SoCs). To achieve this, BrainChip has built a very configurable, event-based neural processor unit that is extremely energy-efficient and has a small footprint. It is complemented with BrainChip’s model compilation tools in MetaTFTM and its silicon reference platforms that can be used by customers to develop initial prototypes and then taken to market.
BrainChip continues to invest heavily in next generation neuromorphic architecture to stay ahead of the current AI landscape. To democratize GenAI, and pave the path to Artificial General Intelligence (AGI)
E.E: What’s the news about new products/services?
N.N: Built in collaboration with VVDN Technologies, the Akida Edge Box is designed to meet the demanding needs of retail and security, Smart City, automotive, transportation and industrial applications. The device combines a powerful quad-core CPU platform with Akida AI accelerators to provide a huge boost in AI performance. The compact, light Edge box is cost-effective and versatile with built in ethernet and Wi-Fi connectivity, HD display support, extensible storage and USB interfaces. BrainChip and VVDN are finalizing the set of AI applications that will run out of the box. With the ability to personalize and learn on device, the box can be customized per application and per user without need of Cloud support, enhancing the privacy and security.
From an IP perspective, the 2nd generation of the Akida IP adds some big differentiators including a mechanism that can radically improve the performance and efficiency of processing multi-dimensional streaming data (video, audio, sensor) by orders of magnitude without compromising on accuracy. It also accelerates the most common use-case in AI – vision – in hardware much more effectively.
E.E: What are the ranges of products/services?
N.N: BrainChip offers a range of products and services centered around its Akida neural processor technology. This includes:
Akida IP: These are BrainChip’s core offerings, representing the neuromorphic computing technology that powers edge AI applications. It has substantial benefits in multi-dimensional streaming data, accelerating structured state space models and vision.
MetaTF: A machine learning toolchain that integrates with TensorFlow, PyTorch designed to facilitate the transition to neuromorphic computing for developers working in the convolutional neural network space.
Akida1000, AKD1500 Ref SoC: A reference systems-on-chip (SoCs) that showcases the capabilities of the Akida technology, and enables prototyping, and small-volume production.
Akida Enablement Platforms/Dev Kits: Tools and platforms designed to support the development, training, and testing of neural networks on the Akida event domain neural processor.
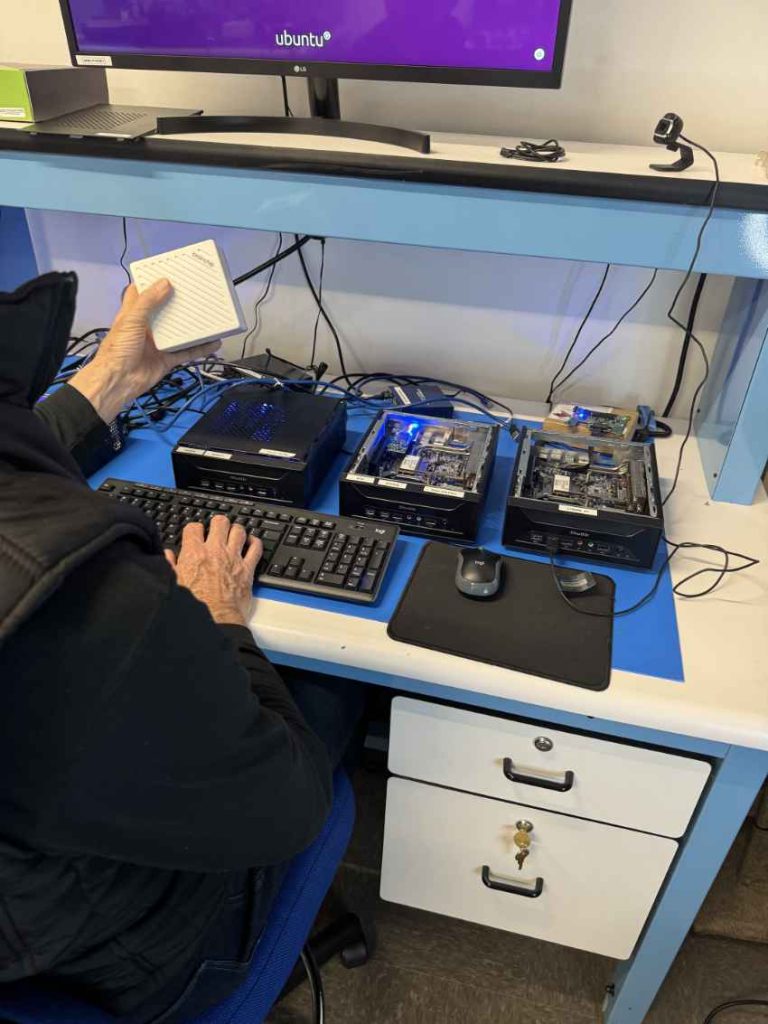
E.E: What is the state of the market where you are currently active?
N.N: We see three different groups of customers in the edge AI industry. The early adopters have already integrated AI acceleration into their edge application and are seeing the benefits of improved efficiency and the ability to run more complex models and use cases.
The second group are currently running AI models on the edge, but they are doing it without dedicated hardware acceleration. They are running on their MCU/MPU. It works but is not as efficient as it could be.
The last group we’re seeing have not yet integrated AI into their edge application. They are trying to understand the use cases, the unique value proposition that AI can unlock for them, and how to manage their data and train models.
We are actively engaged with customers at all three stages and understand the unique challenges and opportunities at each stage.
E.E: What can you tell us about market trends?
N.N: As was evidenced at CES 2024, we’re seeing growth of AI everywhere. For this to be scalable and successful, the growth is happening not just in the data center, but increasingly at the Edge Network and growing to most IoT end devices. We’re at a point where the growth in energy-efficient compute capacity can now run complex use cases like object detection and segmentation on the Edge – not just at the Network Edge, but given technologies like BrainChip’s Akida, even on portable, fanless end-point devices.
By doing more compute at the end point, you can substantially reduce the bandwidth congestion to cloud, improve real-time response and most importantly improve privacy by minimizing or eliminating the transmission of sensitive data to cloud.
Models are becoming larger and more capable but storage and compute capacity at the Edge are constrained, so we see the need for efficient performance, massive compression and innovative solutions take precedence in hardware and software.
Generative AI has a great deal of momentum and it will only become monetizable if there is more done on the Edge. Even on smartphones, there are already thousands of generative AI applications.
There is a clear need to do more with less – which is fundamental to making AI economically viable. The costs include memory, compute, thermal management, bandwidth, and battery capacity to name a few. Customers, therefore, are demanding more power-efficient, storage-efficient and energy-efficient and cost-effective solutions. They want to unlock use cases like object detection in the wild. In addition to limited or no connectivity their use case might require running on battery for months. Traditional MPU/MCU based solutions won’t allow this. BrainChip’s neuromorphic architecture is well positioned for these ultra-low power scenarios.
E.E: What are the most innovative products/services marketed?
N.N: We are seeing great progress in intuitive Human Machine Interface (HMI), where voice-based and vision-based communication with devices is on the rise – in consumer devices, smart home, automotive, remote healthcare and more. For example, automotive is using driver monitoring for emotions, focus and fatigue could help save lives and losses. Remote ECG and predictive vital signs monitoring remotely can also improve not just fatalities but quality of life. AI-driven fitness training is beginning to help individuals stay healthy.
There are lots more.
E.E: What estimations do you have for the beginning of 2024?
N.N: We expect AI to truly go mainstream in 2024, but it’s still the tip of the iceberg.
The big transition you will see is the more mainstream adoption of Edge AI – without it, pure cloud-based solutions especially with Generative AI, would be cost-prohibitive. We therefore see the move towards Small Language Models (SLMs) that draw from (Large Language Models) LLMs to fit better into Edge devices while still providing the accuracy and response time that is expected.
In short, the AI innovation is moving to the Edge, and in 2024, you will see this coming together clearly.