The market for artificial intelligence grew beyond $184 billion in 2024, a considerable jump of nearly $50 billion compared to 2023. This staggering growth is expected to continue with the market racing past $826 billion in 2030. This rapid expansion is driving an increase in the volume of data needed to train AI models, creating new challenges for data management. Effectively handling large datasets requires specialized techniques and approaches that can scale alongside this growth.
Tetiana Verbytska, a technical solution architect at Keymakr, shares insights into her team’s strategies and methods to navigate these complexities. These approaches aim to streamline data workflows, ensure high-quality outputs, and support robust model training across various industries.
Process Optimization and Resource Allocation
Recently, Keymakr was dealing with a unique case related to waste detection. The team received footage of water flowing through a filter, and the goal was to annotate objects such as plastic cups, straws, cigarettes, and other debris passing through.
However, the occurrence of these objects was quite rare. We have a video with 54,000 frames — only about 30 of them would contain debris. Manually checking every frame would not only be time-consuming for a single person, but it would also lead to missing objects due to fatigue.
The team decided to distribute frame annotation among available staff to solve this. The video was split into individual frames and assigned to annotators as static images. Each participant reviewed the pictures and marked those with relevant objects. This approach ensured a comprehensive check of the entire dataset, filtering only the data that contained valuable objects for annotation. It significantly sped up the process, as each person checked a small portion of the video, leaving a selection of frames on which further resources could be focused for detailed processing and annotation.
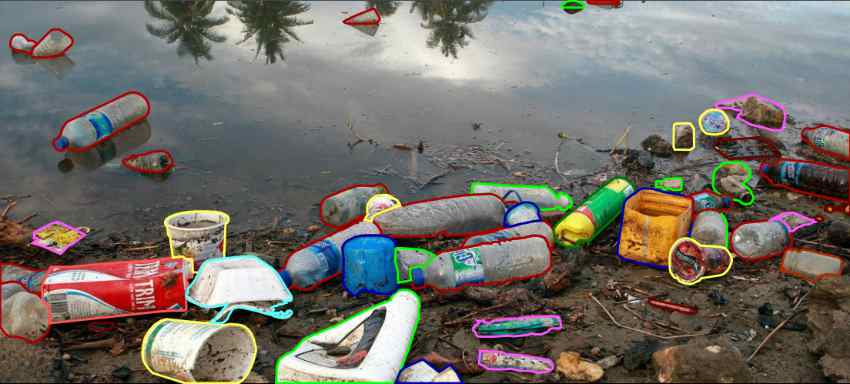
“On average, manual video frame annotation can take up to 5 minutes per image if thorough inspection is needed. This could take months of work for datasets with tens of thousands of frames, so task distribution among the team reduced annotation time by 60-70%,” Tetiana Verbytska says.
Keymakr’s approach shows how providers can use workflows to meet client needs in tough conditions. By leveraging the collective effort of multiple annotators, a labor-intensive task can be turned into a simple, manageable, high-precision process. This method saves time and resources and ensures clients receive accurate, high-quality data.
Data Augmentation and Data Curation for Efficiency
When a client needs a lot of data to improve model training, techniques like data augmentation may be used to expand the data volume. Through this process, the team creates variations of existing frames by altering aspects such as viewing angles, adding blur, or applying vignettes. These modifications help differentiate frames that would otherwise appear identical, infusing each frame with unique visual characteristics. This diversity boosts the model’s ability to learn, and adapt and makes it more robust across different scenarios.
Data augmentation is useful for projects where obtaining new data is challenging or costly. For instance, in medical imaging, collecting fresh images can be time-consuming and limited by privacy considerations.
“Augmentation, therefore, allows us to make the most of the existing data by creating diverse inputs for the model. Beyond technical advantages, it also saves time and resources. Clients can focus on enhancing the data they already have rather than gathering entirely new datasets,” Tetiana explains.
Data curation, meanwhile, enables to prioritize the frames most valuable for the specific needs. It ensures that the dataset aligns closely with the task at hand. Clients in highly specialized fields, like medicine or sports, often rely on pre-sorted images that highlight the most critical data points. For example, radiologists might provide a selection of images showcasing rare but diagnostically important patterns. The curation of the dataset reduces noise and emphasizes quality over quantity.
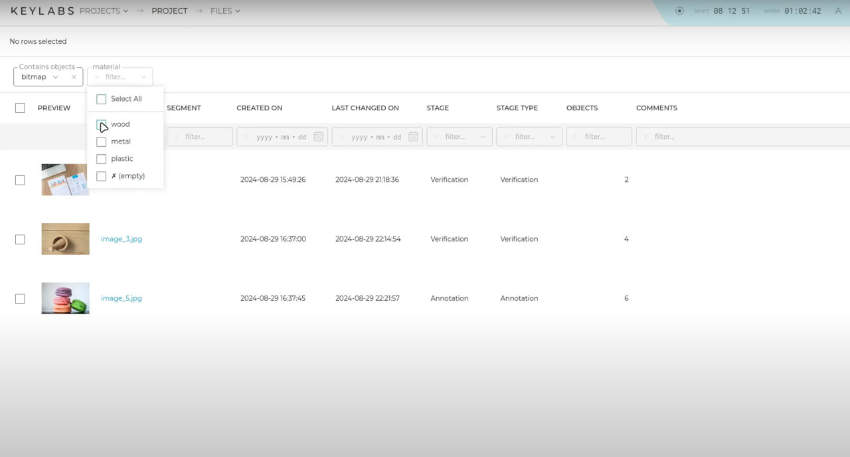
“Data curation is especially invaluable in applications like agriculture, where data volumes may be smaller but still yield meaningful insights. For example, recently our team had a crop monitoring project, where we used a curated dataset. It focused on images capturing early signs of disease and pest presence, maximizing the relevance of each frame,” Tetiana says.
Data augmentation and curation are a powerful combo. It enhances both the breadth and depth of training data, ultimately boosting model efficiency and performance.
Flexibility Based on Project-Specific Requirements
Each industry has unique data requirements. In sports projects, for example, a complete video sequence is essential for tracking movements and building dynamic models. In such cases, clients provide the entire dataset, unfiltered. The goal is to completely capture the performance of every athlete on the field.
In retail, the focus may shift to analyzing customer behavior using in-store surveillance footage. Clients may request specific video segments that highlight customer interactions with products.
However, it is still needed to analyze a lot of data to identify trends effectively. In this case, custom annotation methods are involved. These include object detection and behavioral pattern recognition. They help to sift through large datasets to improve analytics’ accuracy. By meeting these requirements, the team can adapt workflows to the task.
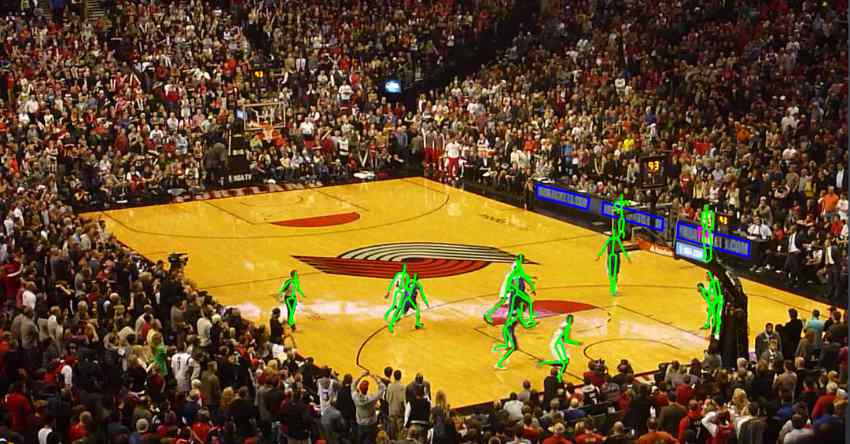
Team and Engagement
“We demonstrate that our team is quality-driven and takes responsibility for the results,” Tetiana says. “This approach is, without exaggeration, one of the most crucial for effectively handling large datasets — especially when it comes to Keymakr’s annotation specialists. They manage each project with utmost seriousness, thoroughly analyzing each case and paying meticulous attention to detail.
Clients deeply appreciate this dedication, particularly when the team asks insightful questions and offers new unexpected solutions. “Commitment to finding tailored solutions and absolute interest in the final results — are the pillars on which our annotation is built“, Tetiana added.