The automotive industry is now the center of a new wave of automation. Smart manufacturing is not just robotics. It is a completely changed approach to quality control, logistics, planning, and energy consumption. The main driving force of this process is artificial intelligence, which must learn to work with “eyes” — cameras, sensors, and IoT systems.
A study published in the journal Sensors found that applying AI to quality control can detect defects early, reduce reliance on manual inspections, and increase detection accuracy. Specifically, AI systems achieve accuracy levels of up to 95–98%, reduce inspection time by 30–50%, and reduce false positives by up to 90%. These figures underscore the impact of AI on the efficiency and accuracy of industrial quality control.
What innovative approaches are used in automotive manufacturing today, and what role does data play in developing robotic systems? Let’s now look into.
Car assembly plants are implementing AI-controlled robots to perform complex and labor-intensive operations, such as body welding, painting, engine assembly, and installation of small components. These robots can be divided into the following types:
- Robotic manipulators (Industrial Robotic Arms) are common in the automotive industry. They weld bodies, apply glue, assemble heavy or large parts, and unload or load objects.
- Cobots. These robots work near people, help assemble small parts, and check product quality.
- Mobile robots. They move components around the shop. Thanks to their sensors, they independently navigate the factory and move loads between work areas.
- Delta robots. Sorting objects and working with small parts. Their speed speeds up routine parts-picking tasks.
An example is the Jaguar Land Rover plant in Halewood, UK, which has undergone a £250 million upgrade. The upgrade included the installation of 750 robots to perform welding, painting, and other operations, as well as autonomous mobile robots for battery assembly.
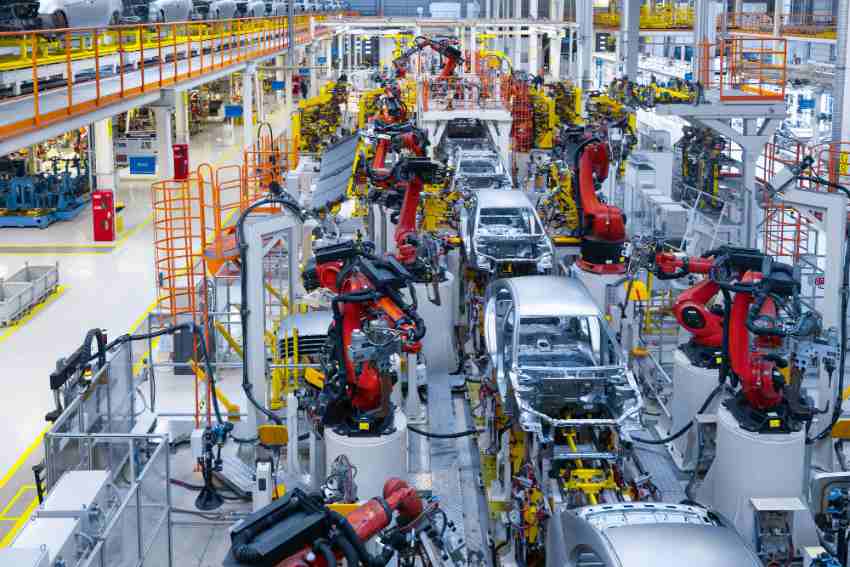
Not Just Control, But Prediction
Another AI approach in automotive manufacturing is predictive analytics, which uses historical data to predict future events or identify potential problems before they occur.
It works on the following principle: first, data is collected (about equipment operation, customer behavior, sales, etc.). Then, an AI model is built based on a mathematical formula or algorithm that learns from this data, and the trained model makes predictions.
For this system to work effectively, it requires a large amount of qualitatively annotated historical data. This includes sound recordings of healthy and defective components, with an accurate mark of the slightest deviations. Such data teaches the AI model to recognize patterns, detect defects early, and predict product quality.
In 2024, Hyundai Mobis implemented a pilot acoustic artificial intelligence system at its Changwon, South Korea, plant. This system analyzes the sounds that occur during the rotation of the electric power steering (EPS). The system can detect defective products at a speed of one unit per second, allowing for real-time quality control. Hyundai Mobis plans to introduce this technology to other production lines, particularly brake systems.
Energy Management
It’s important to remember that AI systems consume a significant amount of energy; however, adopting a sustainable approach is crucial for modern operations. Energy management helps with this. This is an area where artificial intelligence monitors energy consumption in real time at every stage of assembly, from the operation of robotic manipulators to climate systems in workshops. Analyzing large amounts of data, AI identifies inefficient areas, predicts peak loads, and automatically adjusts equipment power. This reduces energy consumption without compromising productivity, reducing electricity costs and CO₂ emissions.
Excellent confirmation of this is Toyota Material Handling’s LTE plant in Italy has been implementing an energy management system by ISO 50001. By 2024, 53 projects had been implemented, including installing smart lighting, real-time energy monitoring, and switching to electric heating. These measures have reduced CO₂ emissions by 710 tonnes per year and increased energy efficiency by 41%.
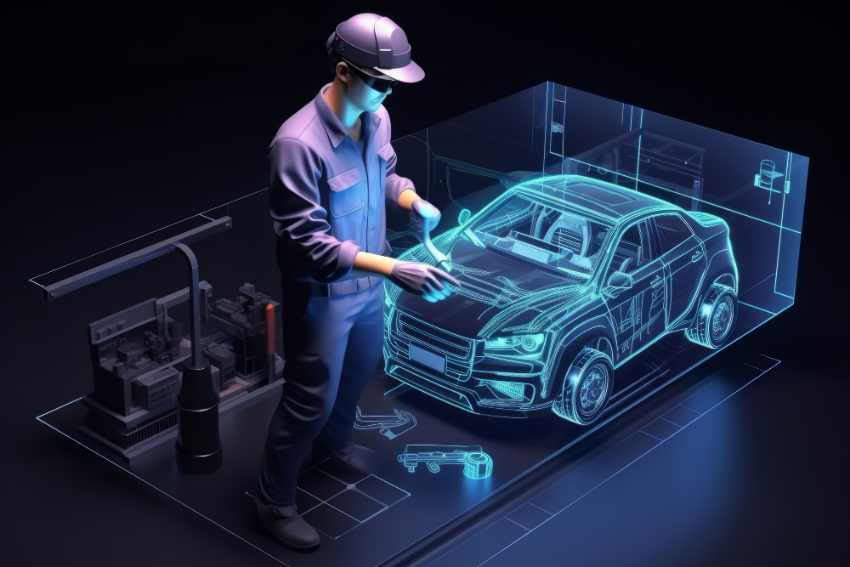
Smart manufacturing is not a replacement for humans. On the contrary, human knowledge and assessment play a key role in the correctness of the annotation in this process. They create the “language” in which AI communicates with machines.
The result is a hybrid system where AI detects a problem, and a human decides to eliminate it or redirect the product.
The effectiveness of this approach is confirmed by the experience of Schaeffler in Hamburg, which implemented the Microsoft Factory Operations Agent system in 2024. Based on large language models, this system analyzes data from production lines to detect defects. However, the final decision on action remains with humans, ensuring a balance between automation and human control.
Where The Data Is, There Is Efficiency
The more complex the product, the greater the annotation requirements. This is especially critical during the factory assembly phase of a car, where cameras and sensors monitor every operation. If a defect detection system is not properly annotated with high-quality, precise data, it can miss damage or deviations, such as incorrect installation of brake hoses or improper placement of batteries in electric vehicles. In such cases, poor data quality can directly compromise the safety of future users.
Quality data is the foundation of any effective AI system in manufacturing.
An annotation provider such as Keymakr offers specialized labeling solutions tailored for manufacturing processes. Depending on the product type and defect characteristics, they apply various annotation types, from polygons to detailed segmentation. This data forms the training backbone for AI systems to work on custom tasks.
For instance, Keymakr experts annotated high-resolution images from the assembly line, labeling issues such as scratches, dents, chips, and paint flaws using polygon and bounding box tools. This annotated data was used to retrain the AI model, whose main task was inspecting products from the manufacturing lines. As a result, the client reduced manual inspection time by 40% and significantly increased defect detection accuracy, highlighting how critical high-quality annotation is to the successful deployment of AI in manufacturing.
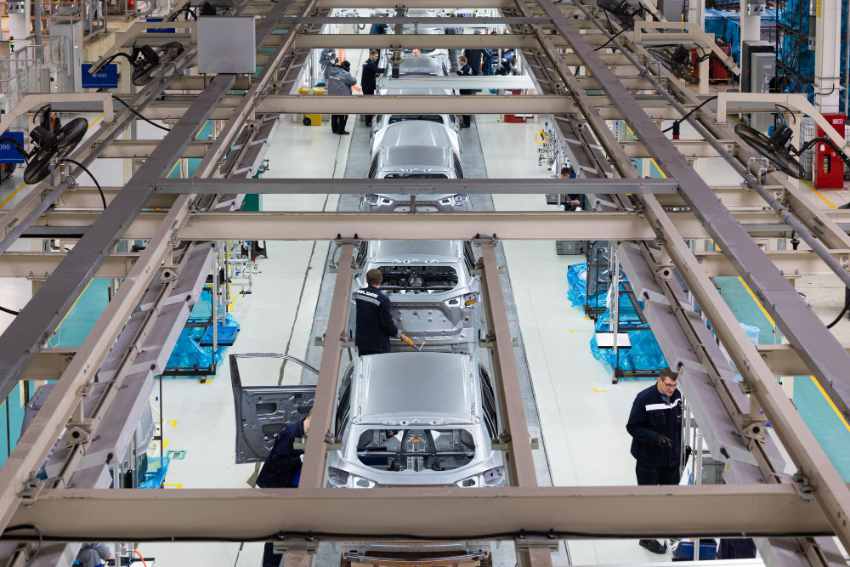
In 2025, large-scale implementation of Edge AI is expected — data processing directly on the factory floor, without transferring it to the cloud. This approach reduces delays in information processing and strengthens cybersecurity since sensitive data does not leave the local infrastructure. Edge AI is especially needed for critical processes, where a few seconds’ delay leads to a decrease in quality or a line stoppage.
Self-learning systems that adjust their parameters based on feedback from production lines are also in focus. For example, if the system detects that a specific equipment setting is increasing the number of deviations, it can adapt its algorithms or change quality control conditions to improve accuracy. In mechanical engineering, this allows for stability and reliability in assembling complex components such as electric drives or power supplies.
Thus, the combination of Edge AI and self-learning systems opens a new era for smart factories with autonomous quality control, adaptation to change, and minimizing dependence on external infrastructure.