The landscape of AI is due for another potential shakeout. Companies like Google, Meta, and OpenAI find themselves in a tough position. They’re running low on high-quality data for ML training – this trend could mean trouble as soon as 2026, slowing down AI innovation significantly.
Publicly available data for teaching AI language models might dry up between 2026 and 2032. Let’s take a look at why and what this may mean:
The Ever-Increasing Demand for AI Training Data
AI technology is progressing fast, causing ML models to grow quickly in both size and complexity. According to a recently published Stanford AI Index Report, model complexity has been growing almost exponentially over the past few years. For illustration, the average Machine Learning model used between 1 and 10 million parameters to interpret data in 2016. Today, these numbers are in the hundreds of millions, reaching 1 trillion for state-of-the-art models.
Here is a handy illustration:
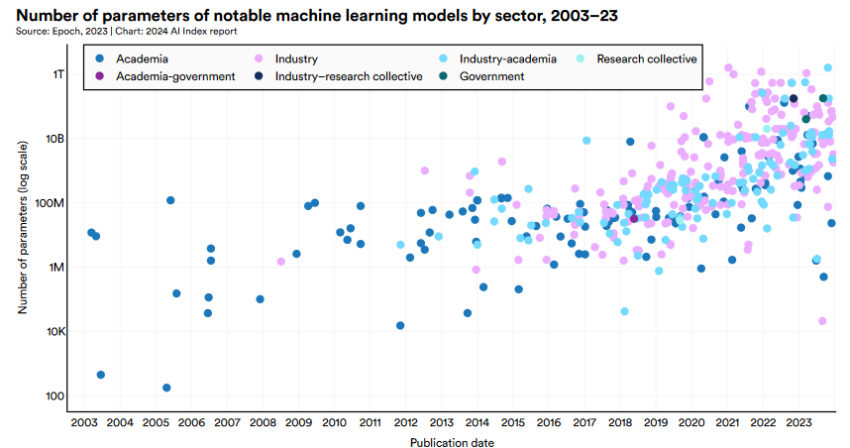
Source: AI Index Report
Models like Gemini, GPT-4, Claude, and others, are all built on staggering amounts of data to do things like understand human languages or see like we do. But pretty soon, there might not be enough data for all the AI training we’re accomplishing. Current sources of all this necessary data might not be able to keep up with the AI field’s growing needs.
This strong desire for data comes from the goal of teaching AI using many different and complete sets of data. This would help close gaps in its knowledge, address biases, and increase the quality of outputs. However, now models are chewing through it at an amazing rate due to the high computation rate and model-training innovations of past years.
Current Sources of AI Training Data
AI companies traditionally use many sources to train their models. They look at web content, online resources, and data from social media. The internet is full of data, like posts and content made by users, blogs, and so on. Open-source datasets, from places like the Linguistics Data Consortium and Kaggle, also play a role. They give AI developers a lot of natural language processing datasets. For Computer Vision systems, we take real-world data like images and videos to then make them readable for machine learning purposes.
Tech companies are always looking for new data sources. For example, Google and Microsoft have access to customer data they often use to train models. However, that data is being processed rather quickly. Looking for creative solutions, some even began reaching out to publishing houses – such as Meta showing interest in buying Simon & Schuster to train AI earlier this year.
Similarly, OpenAI reportedly used over one million hours of YouTube videos to teach its AI to recognize speech. Photobucket is negotiating the prospect of selling 13 billion images to AI companies for model training. The race is heating up as computer scientists aren’t sure if future generations will have enough data to scale and improve models any further.
The Looming Shortage of High-Quality Training Data
AI models are getting bigger and more complex. Yet, the data sources we have are starting to show their flaws. Between 2026 and 2032, AI companies might face a shortage of rich, natural data, a report by Epoch warns.
AI learns from different types of info like texts, sounds, images, videos, and sensor inputs. This could be everyday data from what we do or data specifically created for training to illustrate things like edge cases. Data can also be tagged with labels or left alone, and both ways are useful to some degree. On that note, a lot less of our data is in a training-ready state:
- Structured data is ready for databases and training, tends to be of higher quality, and is easy to analyze.
- Unstructured data doesn’t fit neatly into tables, needs more processing, and takes longer to analyze as a result.
As expected, we create exponentially more unstructured data than structured data:
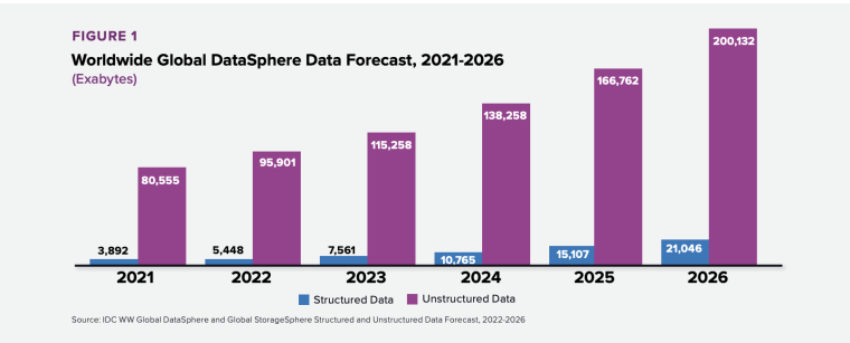
Source: IDC Global
Data Annotation and Labeling Challenges
Labeling and preparing this data to make it structured is tough work for AI makers. It involves picking the right training, checking, and testing data carefully. The data has to be cleaned, put in order, and validated by humans or advanced algorithms so that ML can process it.
When it comes to image annotation, there are many ways to tag data. For computer vision, this can mean bounding boxes, outlining shapes, adding 3D shapes, and marking specific key points. For written words, like in text annotation, you might need to tag names, figure out emotional content, or mark the meaning.For videos, drawing boxes around moving objects and following them is the most common approach. Allthis makes AI capable of ‘seeing’ things and enables everything from self-driving cars to generative AI chatbots.
As AI companies chew through data, they increasingly need to rely on quality over quantity. So, sourcing quality data from specialized companies is one approach they take. However, there are others:
Novel Approaches to Acquiring Training Data
As AI companies look for new ways to gather the data they need, they turn to AI once again, this time to generate the data they can then use to train other models. This is called synthetic or artificial data. Essentially, it’s using generative AI like ChatGPT for text, or DALL-E for images, and then feeding its results to train future generations.
Synthetic data comes in a variety of forms and tends to supplement real-world data. So, for example, someone may synthesize a few hundred hours of voice recordings based on just a few hours of audio. No doubt you’ve seen deepfakes in the past – this would be the equivalent of using them for training AI rather than for hoaxes or memes.
Gartner predicts that a lot of AI training is going to be based on synthetic data in the future:
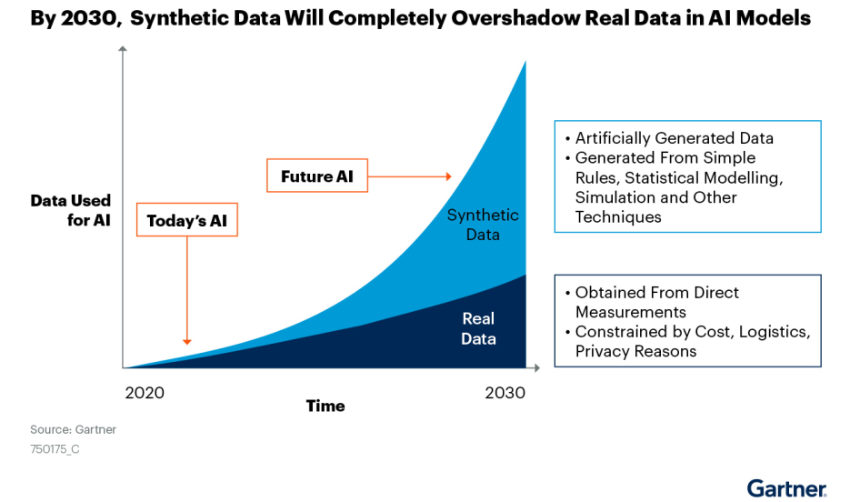
Source: Nvidia + Gartner
Most companies will use advanced AI to craft data for the purposes of training models by 2030.
Challenges of Synthetic Data
Creating synthetic data brings its own issues. It could boost the errors or biases already present in our algorithms. It works like this:
- Generative AI is trained on a biased dataset, let’s say it makes cats red in 85% of cases.
- This model then creates a bunch of other data, among which are a lot of cat pictures.
- Those cat pictures are fed to other AIs that inherit its 85% red cat bias.
- The cycle repeats itself many times over.
Of course, this is a ridiculous example picked to illustrate a real problem. Still, most companies focus specifically on biases and edge cases to mitigate the possibility of this happening. Sometimes they overcorrect, as Gemini has in the past, ironically creating a bias in the opposite direction to the one initially introduced.
In addition, synthetic data is prone to the problem of regression. In essence, the more generations you go through, the more homogenous it becomes. A recent scientific paper cited in the AI index report we mentioned earlier demonstrates this quite well:
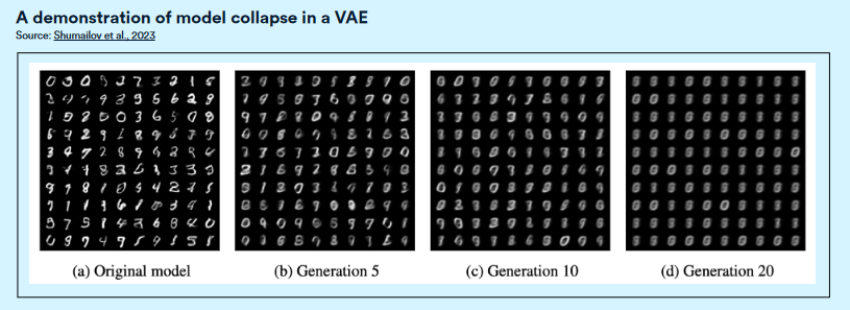
Source: AI Index Report, Shumailov et al
In short, synthetic data isn’t a panacea to the problem of running out of data. Most companies use it to augment real-world datasets rather than for handling most training needs. While models may improve in the future, a method for overcoming this problem of regression is currently unknown.
Still, it’s a fresh path when natural data is hard to find. While synthetic data doesn’t fully tackle the problem, it’s part of the solution.
Data Licensing and Partnerships
Besides making synthetic data, companies are eyeing machine-learning data partnerships. By striking up deals and licensing data, they can get their hands on rare datasets. These datasets might not be open to everyone. This move is crucial for dealing with the absence of high-quality training data. It especially affects areas like conversational AI data, intent classification data, and sentiment analysis datasets.
The AI world is also looking into sharing data more widely. This includes having companies and research teams join forces to make larger, varied datasets. Such a team effort could result in big databases that many in the AI field could use. This might be a good way to deal with the lack of enough data for training, however, proprietary solutions are still preferred by most companies.
Advancements in Data Handling Techniques
Easier ways to label data are being developed. This speeds up the process of getting datasets ready for AI models, turning this vast amount of unstructured data into structured and clean datasets. There’s a rise in automatic or AI-assisted annotation, and in general, quality is overtaking quantity in Machine Learning.
By adopting these fresh ideas and upcoming trends, the AI sector can move towards having a reliable stream of training data. This would help AI grow further. In the evolving AI landscape, securing quality data remains crucial for future innovations.
Ethical Considerations in Data Collection
AI companies are eager to gather more AI training data at any cost, which raises ethical and privacy concerns. In fact, if AI giants want to use your personal information by training, there may not even be many ways of stopping them. Legislators across the globe are beginning to introduce AI laws to understand how to best regulate and handle data better.
When it comes to privacy, In Europe, GDPR lets people delete their uploaded data. COPPA in the US protects kids’ data, outlining strict rules, while HIPAA outlines protected medical information. Respectful and clear data collection is key to keeping the trust of users and protecting privacy rights.
Companies that make smart home gadgets have often faced criticism for overstepping privacy boundaries. They have been accused of collecting voice data without permission. For example, last year Alexa got into trouble for this and agreed to pay the FTC over $25 million, but it’s hardly an isolated incident.
Responsible AI and Bias Mitigation
Making AI fair means finding and lessening biases in collecting data and making decisions. This is important for preventing further inequalities – algorithmic bias occurs due to a lack of good data, or due to skewed datasets. Ironically, it’s in a bit of a push and pull with privacy concerns, because algorithms often get fed only specific data and make biased decisions as a result.
Bringing ethics into AI from the start can reduce harm and improve the situation. AI companies need to carefully step through the ethical challenges of data collection and using conversational AI data. They must stick to the values of privacy, fairness, transparency, and accountability. This is crucial for the honest growth and use of ML.
Summary
The data we use now, like what’s found on websites, in social media, and in public databases, has been great for AI’s growth. However, we’re starting to see it’s not enough. Companies working on AI are looking into new ways to get the data they need. This includes creating synthetic data and partnering with others to get access to more quality sources.
More and more, people are realizing it’s not just about tons of data. They’re focusing on the quality of the data used to train AI models. This helps make the models more accurate without needing models to be endlessly hungry. So, tools like Keylabs are becoming more and more necessary for creating highly specialized datasets and focusing on improving ML models through quality annotation and human-in-the-loop validation.