In-cabin artificial intelligence is rapidly transforming how we interact with vehicles, enhancing both safety and personalization. These systems monitor driver behavior, track passenger presence, and even control the in-car environment.
According to the National Highway Traffic Safety Administration (NHTSA), 94% of motor vehicle crashes in the United States involve driver error. Drivers can make mistakes when fatigued, distracted, or unfocused. AI and accurate in-cabin annotation can help to reduce human mistakes on the road.
Full Automation of Driving
Advanced automotive technologies include autonomous vehicles, advanced driver-assistance systems (ADAS), and personalized in-vehicle experiences. All this uses AI and machine learning to help vehicles understand and adapt to the cabin environment. This makes driving safer, more comfortable, and convenient for everyone.
The ultimate aim for AI in the automotive sector is full automation, where vehicles operate without human input. Achieving this goal will require further development and regulatory changes. Today, the industry is on the brink of realizing Level 3 autonomous vehicles. They can handle most driving tasks but may require human intervention in certain situations. These systems rely on high-quality data, which is made possible through advanced AI training, including in-cabin annotation.
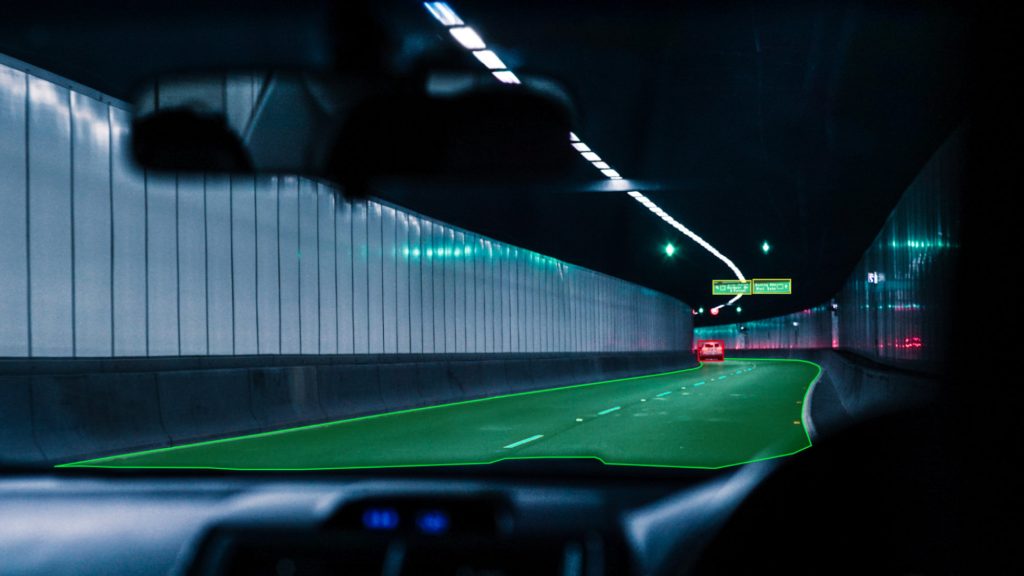
Softs already in use include:
- Driver Monitoring Systems (DMS) uses AI to analyze facial expressions, eye gazes, and head posture to assess driver states and detect distractions or fatigue.
- Emotion detection is essential for road safety as aggressive driving behavior is a safety risk. Automated emotion recognition methods, such as AdaSVM, are being researched.
- Occupant Monitoring Systems (OMS) monitor passengers to optimize safety features like airbag deployment based on occupant size and position.
- Personalized in-vehicle experiences, where AI adjusts things like seat position, climate control, and infotainment to their preferences. This customization enhances comfort and makes the journey more enjoyable for everyone.
How In-Cabin AI Works
In-cabin AI’s core is recognizing objects and understanding human behavior inside the vehicle. Strategically placed systems, from fisheye diagonal cameras to full-on monitoring soft, capture detailed data. AI algorithms analyze this footage in, detecting and tracking passenger activities and driver attentiveness.
Advanced in-cabin AI systems do more than detect objects. They use behavior trees to interpret complex human actions. Behavior trees, used in AI and game design, have nodes for conditions, actions, and decisions. By applying behavior trees to drivers and passengers, AI can understand their behavior and intentions.
DMS: detect and predict
Driving Monitoring Systems are designed to spot distraction, impairment, or fatigue. They analyze facial expressions, eye movements, and head position.
Fatigue is a major cause of accidents, and these systems are key in detecting drowsiness. If a driver starts to fall asleep, the system alerts them, prompting rest.
These systems also serve as early warnings for accidents. They monitor driver actions and alert drivers to risky situations. If a driver looks away for too long or appears incapacitated, the system triggers a response. A study by General Motors and the University of Michigan found that Automatic Emergency Braking reduced rear-end crashes by 46%.
Driver monitoring systems are not just for personal vehicles. Logistics organizations use them to train employees and promote safe driving. By equipping their fleet, managers can track and improve driving habits.
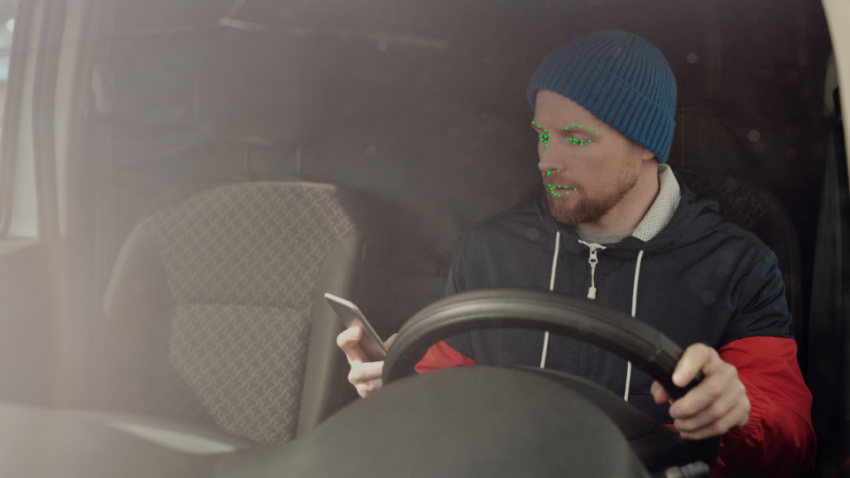
To create effective driver monitoring systems, machine learning models need annotated video and images of in-car behavior.
Creating these annotated datasets involves human annotators labeling each video frame with specialized tools. They track body movements, monitor eye pupil movement, and identify driver emotions.
A recent Keymakr project collected 1,256 hours of video from in-cabin cameras in trucks. Experts annotated various driver activities, using object capturing and recognition, bounding box annotation, and semantic segmentation.
From Object Recognition to Life-Saving Alerts
A key area of improvement in autonomous vehicles is in-cabin object recognition, from personal items to work essentials. AI systems track these items, reminding you to take them when you leave.
More importantly, in-cabin AI can detect the presence of unattended children or pets. If somebody is found, it will send a notification to the driver’s phone. It can also alert emergency services if needed.
Semantic segmentation annotation is key for training AI models to accurately spot and locate objects in images or videos. It assigns a specific class label to each pixel, allowing the AI to distinguish between various objects and their boundaries. With semantic segmentation, AI models can accurately differentiate between objects, even when they are partially occluded or have similar appearances.
Unlike object detection, which uses bounding boxes to identify and localize objects, semantic segmentation provides a more detailed understanding of the scene. It enables the AI to grasp the spatial relationships between objects and their surroundings.
Headway of the In-Cabin Monitoring and Annotation
The automotive industry is on the cusp of significant advancements in in-cabin monitoring and annotation.
Combining data from cameras, radar, and infrared sensors will give vehicles a complete view of the cabin. This will enhance monitoring accuracy, enabling features like driver fatigue detection, occupant classification, and health monitoring.
The impact of these advancements will be profound. Here are some statistics:
- The installation rate of AI-based systems in new cars is expected to increase by 109% in 2025.
- Smart Eye’s software has seen a growth in customer base from 18 OEMs in December 2022 to 21 OEMs by April 2024, with the number of car models equipped with their technology increasing from 194 to 322 during the same period.
Insights from the In-Cabin Conference in Barcelona
Keymakr’s Head of Customer Success, Dennis Sorokin, attended the In-Cabin conference in Barcelona. He shared insights on current trends in the automotive industry. According to him, the greatest demand in the in-cabin technology space is for high-quality data creation. As companies work on innovative solutions, they require real data that is meticulously designed for specific projects.
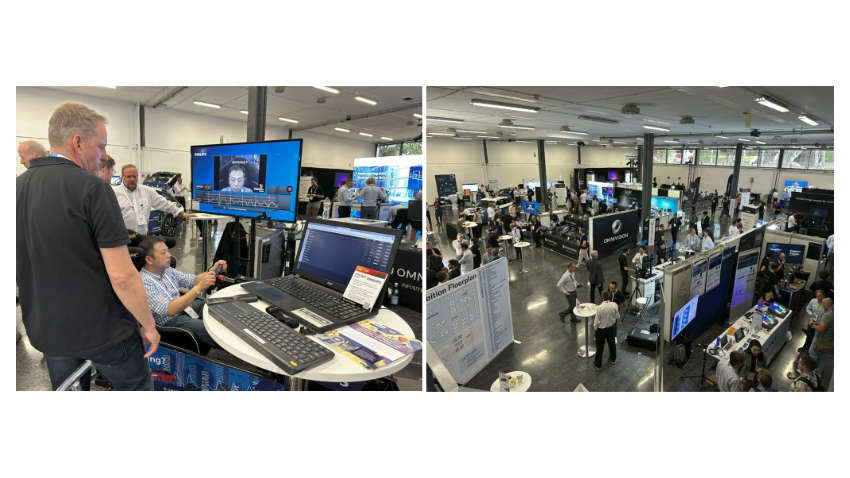
For example, companies like Tesla are focused on improving their autopilot systems.
They already have great results. Tesla’s research says that in the 4th quarter of 2022, they recorded one crash for every 4.85 million miles driven in which drivers were using autopilot technology. For drivers who were not using autopilot technology, they recorded one crash for every 1.40 million miles driven. By comparison, the most recent data available from NHTSA and FHWA (from 2021) shows that in the United States, there was an automobile crash approximately every 652,000 miles.
However, Tesla aims to improve its ability to predict dangerous situations more accurately. To achieve this, it needs to create custom datasets that address highly specific scenarios.
Another situation is when a car company wants to develop AI for a specific category of drivers. Consider a distracted driver. They might be wearing AirPods, have misaligned glasses, and be interrupted by a child in the backseat. Current models may struggle to understand the exact actions of the driver when their hand is off the wheel or interacting with the child.
To improve such models, data must be created to simulate these unique conditions — like detecting a child in the backseat, or recognizing when the driver might be distracted by turning around or performing other actions. The vehicle’s sensors, radars, and thermal signatures can work together. However, custom datasets are vital to training models for these scenarios. This allows the car to prepare for braking or heightening attention to its surroundings.
Keymakr specializes in creating and delivering real custom datasets tailored to the most demanding products on the market. It helps companies solve complex challenges and implement innovative projects directly.