The growing use of image data annotation tools in the automotive, retail, and healthcare sectors is powering rapid market expansion. In healthcare, especially, there is a high demand for accurate annotation to turn vast amounts of data from various devices and applications into actionable diagnostics. As Roots Analysis says nearest years most of the AI medicine solutions (42%) are being used for analyzing CT images, followed by those employed for processing MRI (24%), X-ray (21%), and ultrasound images (16%)
Recently Keymakr annotation company and Keylabs platform have been recognized as key players in the Medical Image Annotation Software market. Let’s examine the features, challenges, and complexities involved in medical annotation, and explore how annotation companies address these issues using Keymakr insights.
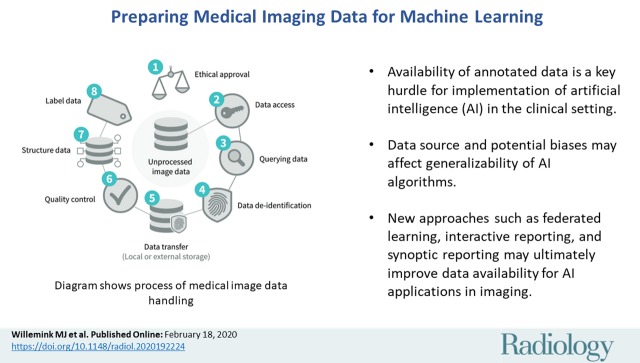
Effective Strategies for Medical Data Preparation
Diverse datasets are key to success in medical imaging ML models. To ensure reliability and avoid biases, it’s vital to source data from various locations, check for errors, and maintain data quality assurance. An 80-10-10 split for training, validation, and testing improves model testing and refinement.
In medical data preparation, quality trumps quantity. A smaller, well-maintained dataset is more valuable than a large, inconsistent one. This ensures that machine learning models produce accurate and reliable results, which is critical in healthcare.
To create a representative dataset, Keymakr advises these strategies:
- Collect data from diverse sources, including various geographic locations and demographics.
- Ensure data is accurately labeled and annotated by domain experts.
- Implement rigorous quality control measures to identify and correct errors.
- Maintain a balanced distribution of classes within the dataset.
- Regularly update and expand the dataset to keep pace with medical knowledge and technology advancements.
The Technical Challenges Unique to Medical Images
Medical images are more complex than everyday pictures, presenting several technical challenges. For instance, images from modalities like X-rays, CT scans, MRIs, and ultrasounds each have unique characteristics. Annotation tools must be robust to handle diverse data formats and ensure compatibility across different systems
The main differences in medical images from others include:
- Layer complexity: Medical images often have multiple information layers. They may show different tissue types or anatomical structures, which need to be accurately identified and labeled.
- Image size and resolution: Medical images can be much larger and higher-res than standard ones. They need special tools and methods for efficient processing and annotation.
- Bit depth: Medical images often have higher bit depths. They allow for a greater range of grayscale or color values. This can affect the accuracy and complexity of the annotation process.
As an example, a medical research organization worked with Keymakr using the Keylabs platform. They trained an AI model to detect cardiovascular disease from ultrasound scans. Ultrasounds present unique challenges. They are dynamic, showing real-time sequences and displaying multiple tissue layers. Subtle movements in them can indicate health issues.
Keylabs fixed this by allowing frame-by-frame annotations of heart structures, like chambers and valves. Custom tools allowed precise marking of blood flow regions. A built-in quality control workflow lets medical experts review and refine annotations, ensuring clinical accuracy. This setup improved the model’s early disease detection rates by 25%, accelerating diagnosis and patient care.
Insights into Different Types of Labeling Tasks
Medical imaging employs various labeling tasks to annotate and classify data for AI and machine learning. These tasks span from simple classification to complex segmentation.
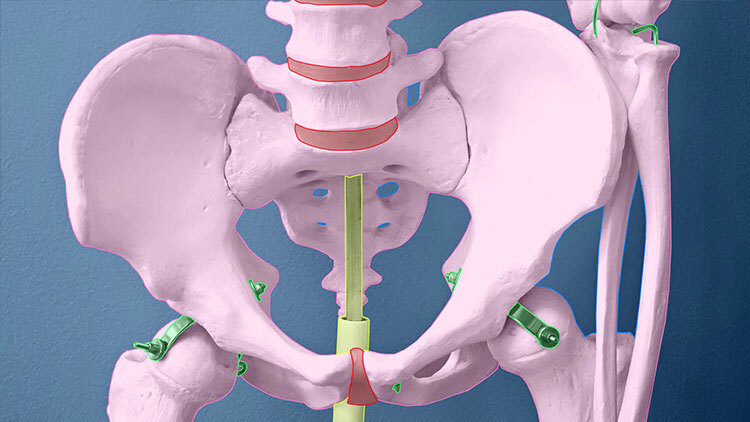
Classification is a fundamental task, assigning labels to medical images based on visible features. It categorizes images by factors like disease stage, or anatomical structures. Accurate classification helps AI models find patterns. This aids in diagnosis and treatment.
Object detection identifies and localizes specific objects in medical images. It’s vital for analyzing scans like CT or MRI, focusing on structures like tumors or organs. Object detection helps AI find areas of interest. It aids in accurate diagnoses and treatment plans.
Segmentation divides medical images into segments or pixels belonging to different classes. It includes semantic segmentation and instance segmentation. Semantic segmentation labels each pixel, enabling detailed tissue and structure analysis. Instance segmentation distinguishes individual objects of the same class. It is essential for complex cases, like multiple tumors in one scan.
Landmark detection, or keypoint annotation, identifies specific points or landmarks in images. It aids in precise measurements, anatomical studies, and disease localization.
As an example, Keymakr collaborated with an orthopedic research facility to improve AI models analyzing X-rays for joint diseases. Using landmark detection, Keymakr annotators marked specific points in images, such as bone edges and joint centers. This key point annotation enabled the model to accurately measure distances and angles, helping clinicians track disease progression and plan surgical interventions.
Special Requirements for Annotators’ Expertise
Annotators working on medical data annotation projects need specialized expertise. This expertise goes beyond general data annotation skills. It includes:
- Medical knowledge: Annotators must have a strong understanding of human anatomy, physiology, and various disease states. They must identify and label relevant features in medical images.
- Familiarity with medical terminology: Medical images often use complex terms and abbreviations. Annotators must understand and interpret them correctly.
- Knowledge of imaging modalities: Annotators should know medical imaging types, like X-rays, CT scans, and MRIs. They should understand the unique traits and challenges of each.
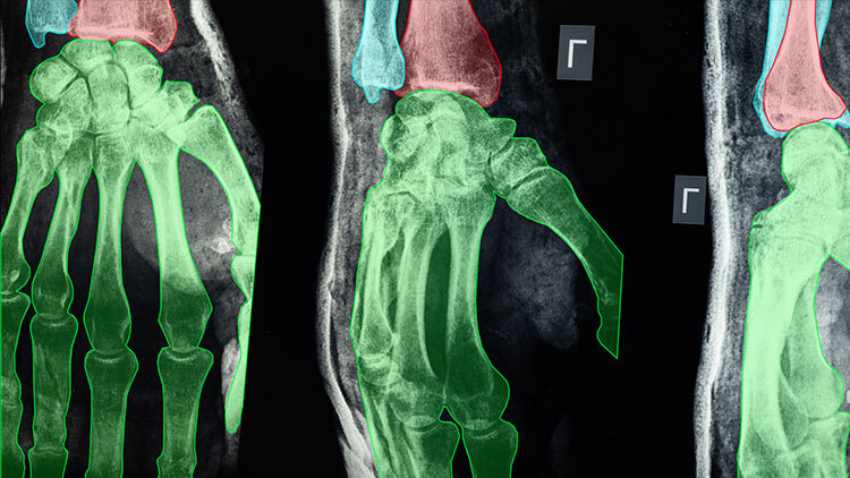
For instance, Keymakr’s in-house teams include certified radiologists, pathologists, doctors, and medical specialists, as well as trained medical students and domain experts focused on medical annotation.
Using automatic annotation for routine tasks, Keymakr always engages expert annotators to check and validate the medical data. Human data curation is critical for ensuring dataset integrity, compliance, and quality. It involves reviewing and validating annotated data, resolving errors or discrepancies, and storing data in a suitable format for machine learning.
The Biasness Challenge: Hurdle for AI in Healthcare
Collecting high-quality X-ray, CT, and MRI data for medical AI can be tough. There are technical issues and biases in clinical data.
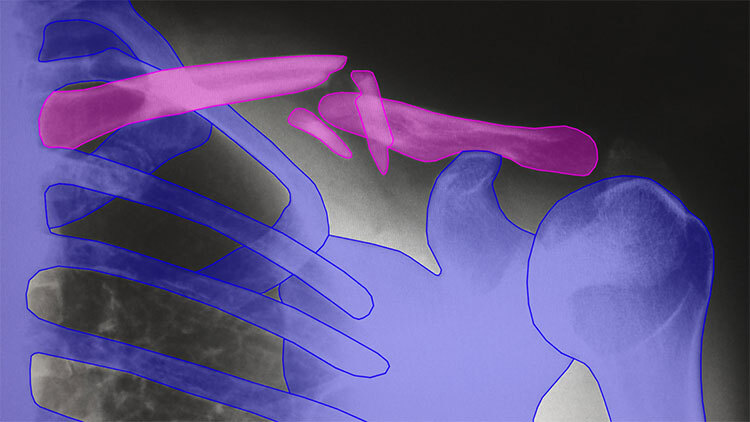
Data biases stem from historical healthcare disparities and unequal access to care. Many datasets overrepresent some demographics. AI models trained on this data are less accurate for underrepresented groups. This bias can result in misdiagnoses or inappropriate treatment recommendations.
Keymakr addresses these challenges and mitigates these biases by partnering with local and overseas partners. Keymakr builds custom medical imaging datasets understanding the nuances of healthcare data collection and annotation.
For companies without a defined process, Keymakr can help design a tailored approach that ensures datasets are representative and ethical, promoting equitable healthcare outcomes.