In today’s world, personalization is no longer a luxury — it’s an expectation. AI-driven personalized content recommendations are reshaping how audiences interact with digital platforms. From curated playlists on Spotify to tailored movie suggestions on Netflix, AI-powered systems are reshaping user experiences and driving business outcomes.
Let’s explore how AI-powered personalization works and its growing significance, particularly through the lens of Computer Vision — a field where annotated data plays a vital role in success.
Understanding AI-Powered Personalization
Personalization powered by AI revolutionizes content delivery. It uses machine learning algorithms to analyze vast user behavior data, identifying patterns and preferences to recommend relevant content. This technology has come a long way, evolving from basic profile-based systems to dynamic, real-time personalization engines that adapt to every click, swipe, and interaction.
AI-driven personalization has become a critical strategy for businesses aiming to enhance user engagement and boost revenues. The global AI in media & entertainment market size is expected to reach USD 99.48 billion by 2030, registering a CAGR of 24.2% from 2025 to 2030. This growth reflects the increasing demand for precise, tailored content delivery.
For companies, the appeal lies in enhanced efficiency and user satisfaction. Personalization minimizes irrelevant content, ensuring users receive recommendations that match their preferences. Platforms utilizing AI capabilities report tangible benefits, including higher conversion rates, improved retention, and reduced operational costs.
AI-powered recommendations simplify the overwhelming process of content discovery. By offering curated suggestions, these systems save users time and effort, creating an intuitive browsing experience.
Key features include:
- Real-Time Suggestions: AI responds instantly to user actions, ensuring relevance.
- Optimized Interfaces: Tailored layouts cater to individual preferences.
- Content Categorization: Data-driven insights enable precise content grouping based on taste and behavior.
- AI systems gather extensive user data to power their recommendations. This encompasses:
- Viewing patterns
- Time spent on content
- User ratings and reviews
- Search queries
Through this data analysis, AI can categorize users based on similar tastes, enhancing the accuracy of content suggestions. Netflix, for example, conducts about 250 A/B tests annually on 100,000 users to enhance its recommendations.
The Role of Computer Vision in Personalization
While much of AI’s personalization involves text and behavior analysis, Computer Vision takes this capability to the next level by interpreting and acting on visual data. As a data annotation company, Keymakr emphasizes the importance of labeled visual datasets in training these advanced systems.
Netflix: Pioneering Visual Personalization
Netflix employs Computer Vision to analyze its extensive media library, tailoring recommendations based on visual cues. Key techniques include:
- Dynamic Thumbnail Selection: By identifying engaging frames, Netflix customizes visuals to resonate with individual users. For instance, if a user shows interest in a specific actor, the thumbnail might highlight that actor prominently. By testing different thumbnails, Netflix identifies which visuals drive more clicks and engagement.
- Genre and Mood Classification: Netflix decodes visual features such as color palettes, scene composition, and actor expressions to classify content by genres or mood (e.g., romantic, suspenseful). This metadata enhances its recommendation algorithms, making suggestions more tailored to individual tastes.
- Enhanced Search: Visual understanding of content allows users to search for “shows with fight scenes” or “movies with landscapes,” relying on the system’s ability to recognize such elements in videos.
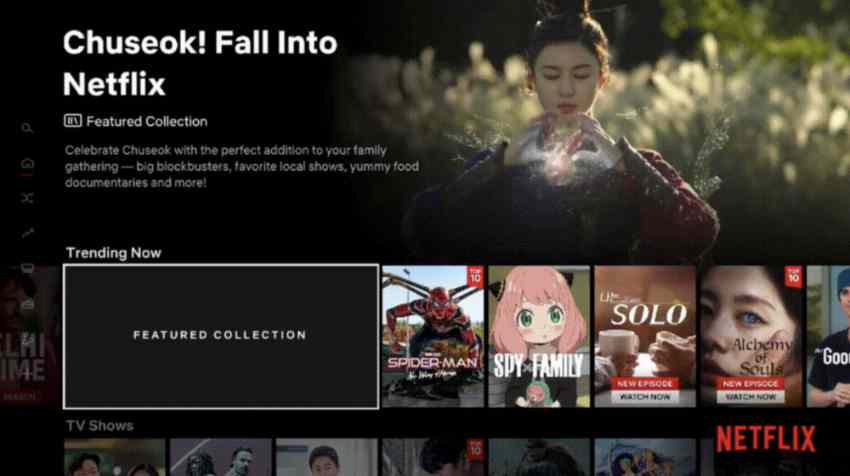
Spotify: Merging Visuals with Audio
While Spotify primarily deals with audio content, Computer Vision contributes to enhancing user engagement through visual content associated with music and podcasts.
Album Art Analysis: Analyzing album art, which often carries clues about the music’s genre, tone, or era, helps the platform recommend playlists or albums with similar aesthetic styles to users.
Video-Based Recommendations: Short video loops and “Canvas” features, integrating visual storytelling with songs are analyzed to recommend visually similar content, creating a richer multimedia experience.
Retail: Enhancing Product Recommendations
In e-commerce, Computer Vision helps retailers analyze product images to deliver tailored recommendations:
- Visual Similarity Search: Platforms like Amazon and Zalando use computer vision to suggest products similar in style, color, or design to those a user has browsed.
- Dynamic Styling Suggestions: Fashion retailers leverage image recognition to create personalized outfit recommendations based on customer preferences and browsing history.
- AR Shopping Experiences: Augmented reality tools powered by computer vision enable users to visualize products in their environment, such as trying on virtual clothing or placing furniture in a room.
Artificial intelligence is revolutionizing website discoverability, helping consumer brands break through the noise and match their customers with the right products faster than ever. The best of these technologies can be observed in action at NRF Innovators Showcase at NRF 2025: Retail’s Big Show, which Keymakr will also attend.
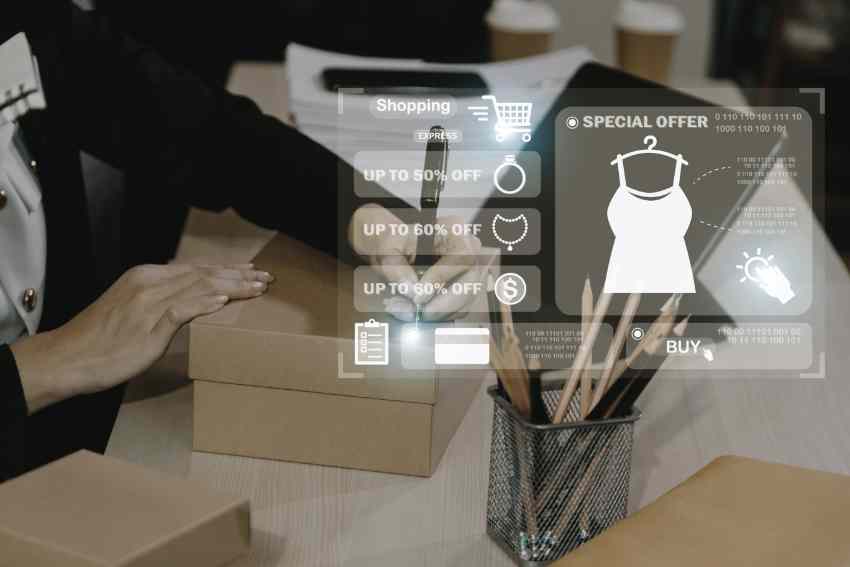
Social Media: Powering Visual Content Engagement
Social media platforms utilize computer vision to deliver content that resonates with individual users:
- Content Curation and Discovery: Instagram’s Explore page and Pinterest’s visual search rely on computer vision to recommend images, videos, and posts similar to a user’s preferences.
- Ad Targeting: Platforms like Facebook analyze images and videos to display ads that align with user interests. For example, if a user frequently interacts with images of outdoor gear, they are likely to see advertisements for hiking equipment.
- Automatic Content Tagging: Tools like image recognition allow platforms to categorize photos and videos, making it easier for users to discover relevant content.
AI personalization has become a major privacy concern in our digital world. With GDPR compliance tightening, companies must focus on protecting data and gaining user consent. It’s essential to have clear data usage policies to build trust and avoid legal issues.
Companies should give users the option to opt out of AI features. Setting ethical AI guidelines and using human oversight can reduce privacy risks while keeping users’ trust.
As the demand for personalized content continues to rise, the collaboration between advanced AI models and expertly annotated data will only deepen. Computer vision, in particular, will unlock new possibilities, from more intuitive media recommendations to immersive user interfaces. By combining technical expertise with high-quality human-verified data, companies can take advantage of this powerful market opportunity.